Applications of Artificial Intelligence in the Pharmaceutical Industry and Research
Updated on: April 06, 2024
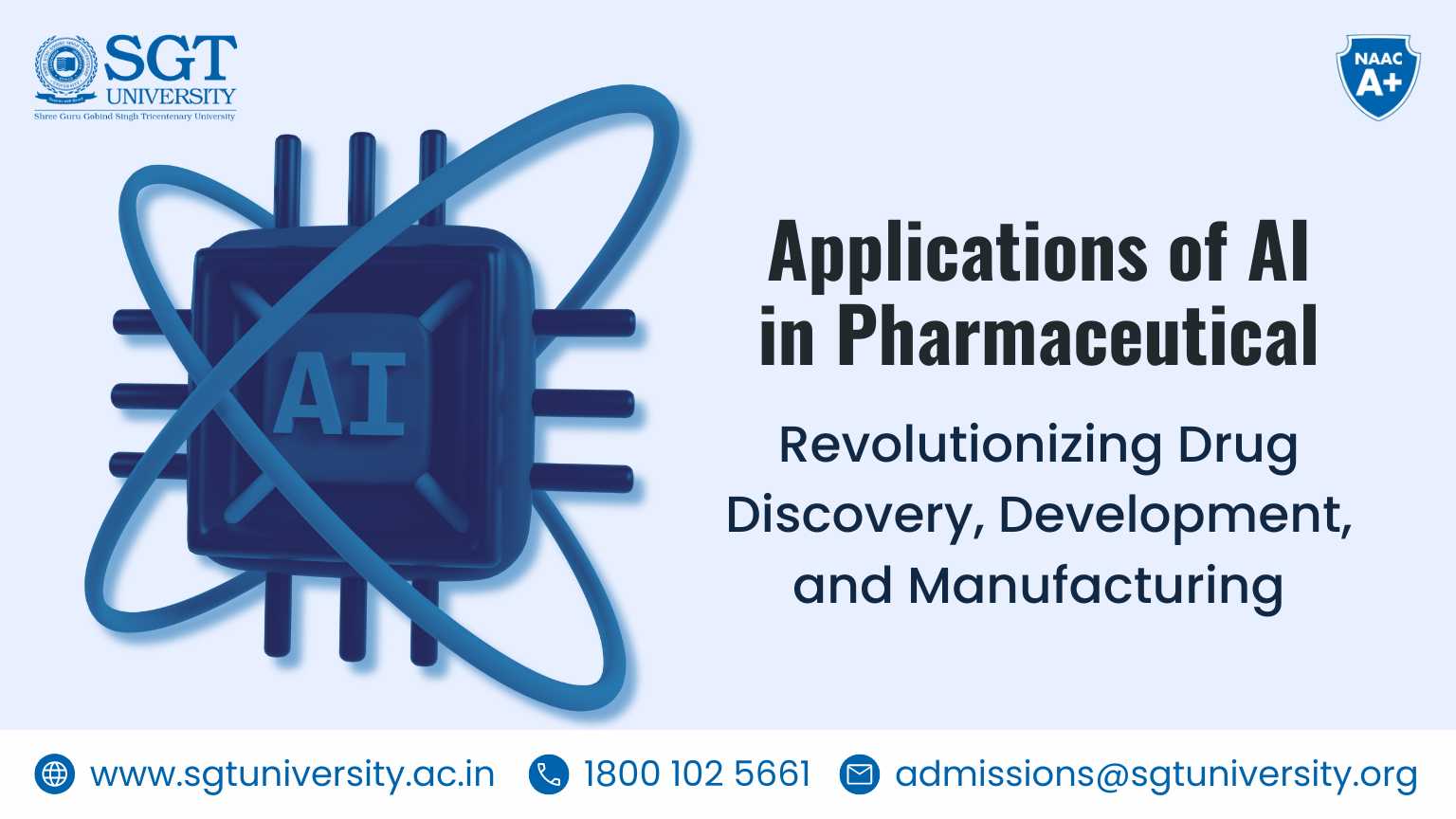
Leveraging AI in Pharma: Revolutionizing Drug Discovery, Development, and Manufacturing
Artificial Intelligence (AI) is becoming more and more prevalent in several aspects of society, especially in the pharmaceutical industry. AI has benefits both professionally and economically. Minimizing the need for human labour, it mimics human intelligence and combines it with state-of-the-art technology to produce the finest outcomes. In the pharmaceutical sector, digitalization has become increasingly prevalent. One of the challenges that change invariably brings, is the requirement for scrutiny and reliable information to handle matters pertaining to its acquisition and implementation in the field. Drug delivery is a field that is currently growing at a faster rate than pharmaceutical sciences, biosciences, and engineering combined. The development of computational techniques that facilitate the design, engineering, and production of nano systems has led to this extraordinary shift. Drug delivery nano systems' design, characterization, and production stand to benefit greatly from artificial intelligence (AI). Furthermore, the ability to perform reverse engineering and ongoing system optimization is becoming possible with the use of big data.
AI is used to facilitate the planning, execution, and recruitment of patients for clinical trials. It is frequently combined with enhanced patient monitoring during clinical trials, or with medical devices accessing personal patient data to guide medical decisions. DSP-1181 is reportedly the first medication of this kind developed with AI to reach clinical trials. In contrast to four years utilizing traditional procedures, Ex Scientia, which developed DSP-1181 in collaboration with Sumitomo Dainippon Pharma of Japan, noted that it had taken less than 12 months from the start of preclinical testing to its conclusion (Sumitomo Dainippon Pharma 2020). Thanks to the pharmaceutical industry's optimization of AI, new drug research is moving along at an incredibly fast pace. Also, a potent new medication that can eradicate numerous species of bacteria resistant to antibiotics is found by an AI model. The computer model is intended to identify possible antibiotics that kill bacteria by methods distinct from those of currently available medications. It can screen over 100 million chemical compounds in a matter of days. In general, the use of AI in pandemic control has demonstrated significant promise in several areas, including as tracking patients, stratifying asymptomatic patients, anticipating epidemic trends, and identifying possible repurposing medications. Although AI offers enormous potential for improving medicine delivery and discovery, but it also has significant drawbacks that eventually necessitate human intervention or the need for experts to understand the intricate outcomes. The datasets provide most of the AI predictions; but, because of the Gray area in the results, human interpretation is necessary to arrive at the right conclusion. When processing data for predictions and evaluating hypotheses, AI may encounter problems with algorithmic bias. Furthermore, finding inactive molecules because of docking simulations is a regular occurrence. Therefore, to effectively make decisions and do cross-verifications to rule out system bias issues, a careful review of these factors still requires human input.
Depending on the type of data in a particular problem domain, supervised learning problems can be solved using a variety of approaches. Naïve Bayes, K-nearest neighbours, support vector machines, random forest, ensemble learning, linear regression, support vector regression, and other methods are some of these approaches. As outlined below, it has several uses in the pharmaceutical sector:
- Drug Design and Discovery: The features or activities of novel drug candidates can be predicted through the application of supervised learning algorithms. The model may identify patterns and connections between desired outcomes and molecular properties by training on a dataset of known substances and the actions that go along with them. This helps in drug discovery and design by enabling the prediction of novel compounds' activity, potency, or toxicity.
- Predictive maintenance and quality control: Supervised learning can be applied to pharmaceutical manufacturing to support both processes. The model can be trained to forecast equipment failure, product quality deviations, or process anomalies using data from production processes, equipment sensors, or quality testing results. This enables proactive maintenance and quality assurance.
- Drug Target Identification: By examining biological data, supervised learning algorithms can assist in the identification of possible drug targets. The model can learn patterns and indicate possible targets for more research by being trained on data pertaining to genomic, proteomic, or transcriptomic traits and their relationship to treatment response or illness progression.
- Prognosis and Disease Diagnosis: Based on medical data, supervised learning models can be used to forecast patient outcomes or make disease diagnoses. The model can predict the course of the disease or the response to treatment by training on labelled datasets that comprise patient characteristics, clinical data, and disease outcomes.
- Adverse Event Detection: Pharmacovigilance data can be used to identify and categorize adverse events linked to medications using supervised learning algorithms. The model can be trained using labelled adverse event data to find patterns and potential safety signals, which will aid in the identification and characterisation of adverse occurrences.
- Clinical Trial Predictive Modelling: Clinical trial outcomes can be predicted through the application of supervised learning. The model can be trained to predict patient response, treatment efficacy, or safety outcomes using historical clinical trial data, which includes patient characteristics, treatment interventions, and trial outcomes. This data can improve patient selection and serve as a guide for trial design.
These are but a few instances of the pharmaceutical industry's use of supervised learning through AI. In many phases of pharmaceutical research, development, and manufacture, supervised learning approaches, when paired with suitable feature selection, data preprocessing, and model evaluation, can offer insightful information and facilitate decision-making.
Blog by: Ms. Arshnoor Kaur
Supervised by: Dr. Sushama Maratha, Dr. Manvi
Editor: Prof. (Dr.) Vijay Bhalla
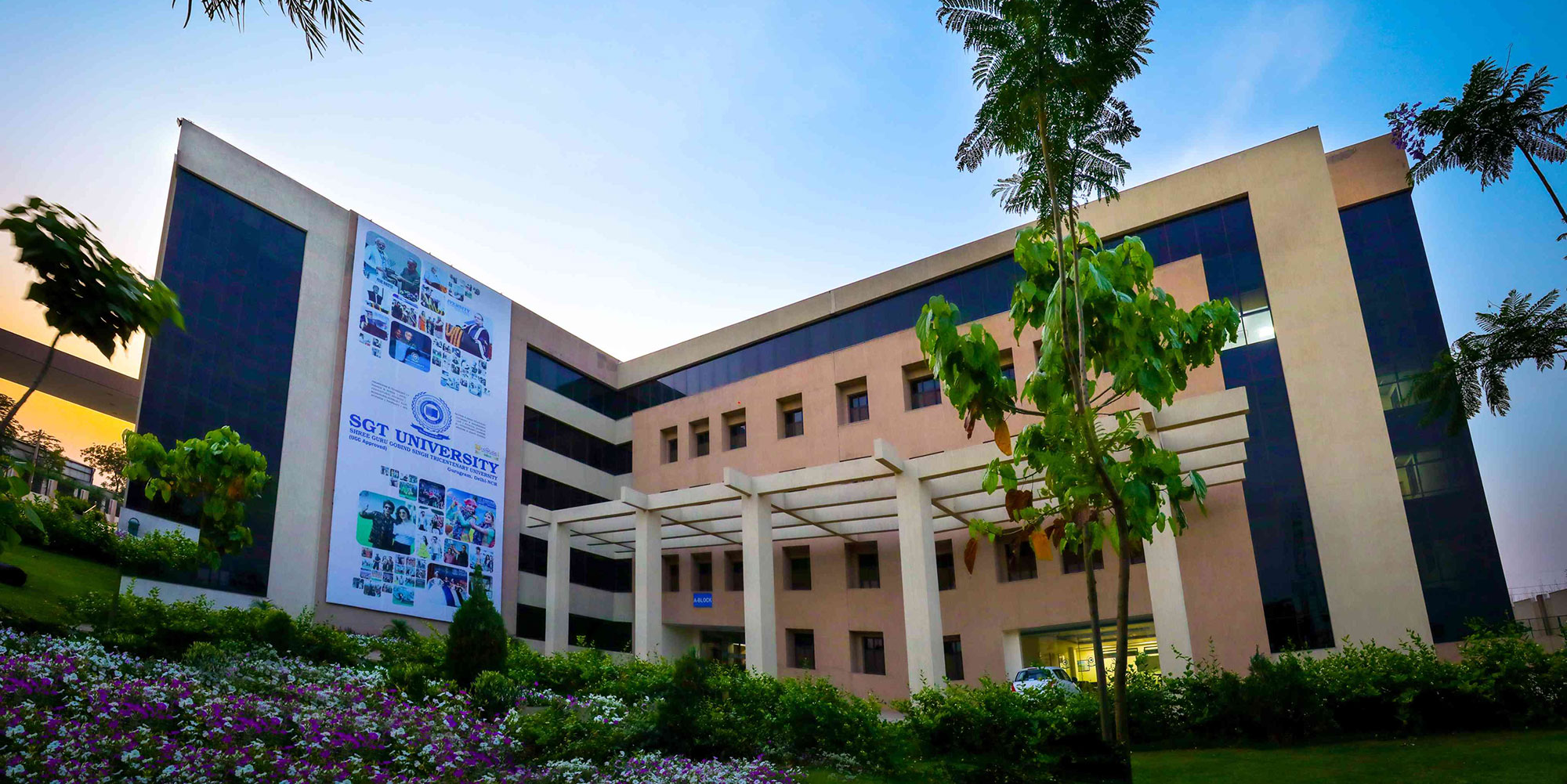